GCP Pub/Sub Integration
To configure the GCP Pub/Sub integration, complete the following steps.
Follow the steps to Connect a Device. The device will be used to store tags that will be eventually used to create outbound topics in the connector. Make sure to select the Enable Data Store checkbox.
After connecting the device in Litmus Edge, you can Add Tags to the device. Create tags that you want to use to create outbound topics for the connector.
To add the Kafka connector in Litmus Edge:
- Navigate to Integration.
- Click Add a connector icon. The Add a connector dialog box appears.
- Select Google Cloud Pub/Sub provider from the drop-down list.
Complete the following information for the Google Cloud Pub/Sub connector as shown in the screenshot and click Update.
- Click the Google Cloud Pub/Sub connector tile. The connector Dashboard appears.
- Click the Topics tab.
Click the Import from DeviceHub tags icon and select all the tags to send data to Databricks.
In Databricks, you can configure GCP Pub/Sub connector parameters from a Python notebook file. You need to authenticate using service account credentials and specify Pub/Sub topic.
To set up Databricks for GCP Pub/Sub Streaming:
1. Navigate to Databricks workspace using your log in credentials.
2. Create a new notebook in Databricks. Follow the Databricks Pub/Sub Streaming Guide to set up Google Pub/Sub streaming.
3. Once the data is loaded, it will be in the following format:
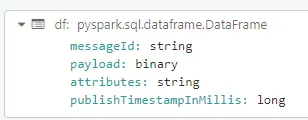
The payload sent by LE will be in the payload field in binary format.
Note: The user can create their own notebooks and customize them as needed. This example notebook will explain how to ingest the standard DeviceHub JSON Payload.
Open a new notebook and follow the steps:
1. Import the necessary libraries and dependencies to handle Pub/Sub data streams and process data using PySpark.
2. Define the credentials and GCP Pub/Sub information that will be used to authenticate and connect to the Pub/Sub service.
Note: End-users should use secure methods of passing their Pub/Sub credentials.
3. Configure Spark to read data streams from the Pub/Sub topic.
Note: Ensure to include the subscription ID, topic ID, project ID, and authentication options.
This will output the following:
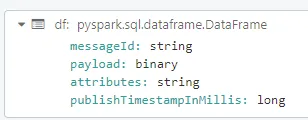
Note: This guide covers the DeviceHub JSON Payload. Please update the notebook if you are sending a different payload to Databricks.
4. Define the schema that matches the expected structure of the incoming data and parse the Pub/Sub stream data accordingly. This involves decoding the payload, extracting attributes, and structuring the parsed data into a DataFrame.
5. Once the data is published to the Databricks table, you can query the table to verify that the data ingestion process is successful.
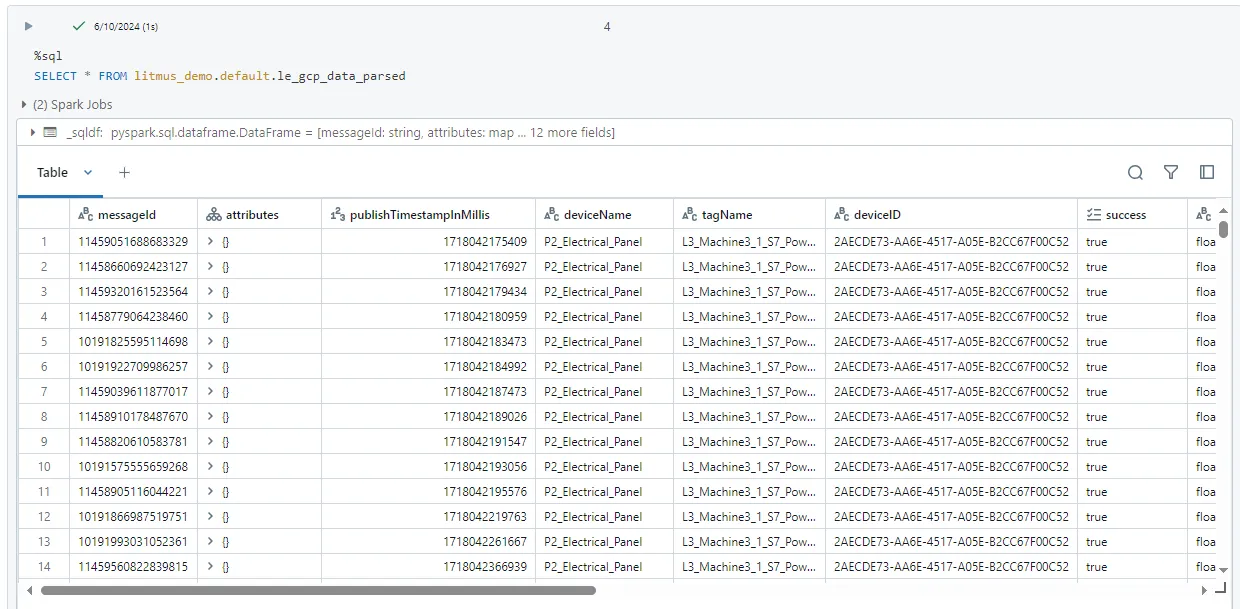