Use the Signal Decomposition Function
You can use the Signal Decomposition function to extract and separate signal components from composite signals, which are related to semantic units. This function decomposes signals by computing trends through linear regression and seasonality through naive differencing.
Review the following scenario for the Signal Decomposition function. Then, you will simulate PLC data and calculate the trend in the data.
In a wind farm, the Signal Decomposition function is utilized to analyze the output from turbines. It helps to separate the consistent patterns due to seasonal wind changes from the overall trends in turbine performance. This information is crucial for scheduling maintenance during expected low-wind periods and optimizing energy production throughout the year.
Follow the steps to Connect a Device and configure the following parameters:
- Device Type: Simulator
- Driver Name: Generator
- Enable Alias Topics: Select the checkbox.
After connecting the device, add the following tag. See Add Tags to learn more.
- Name: Select S - Random value generator
- Value Type: Select float64
- Polling Interval: Enter 1
- Tag Name: Enter input1
- Min_value: Enter 101
- Max_value: Enter 199
You can now create the analytics flows using data from the device and tag you previously created.
To create an analytics flow with the Signal Decomposition Processor function:
- In Litmus Edge, navigate to Analytics.
On the analytics canvas, click Add processor. The Create a processor dialog box displays.
- Select DataHub Subscribe.
In the Topic field, click the Search icon, select the device you previously created, and then select the alias topic for the input1 tag.
- Click Save.
- Click Add processor again and select the Signal Decomposition processor. The Edit a Processor dialog box appears.
- Window Size: Enter a value that represents the range to apply the signal decomposition function. For this example, we input a value of 10.
- Model Type: Select between additive and multiplicative.
- Additive model: Signal = Trend + Seasonality + Residue
- Multiplicative model: Signal = Trend * Seasonality * Residue
- Periodicity: Enter a value to determine how frequently the observations are spaced in time. For this example, we input a value of 5.
Click Save.
- Connect the DataHub Subscribe processor (tag: input1) to the Signal Decomposition processor with a wire and use the events connection.
- On the analytics canvas, click Save. The configured analytics flows should look like the following:
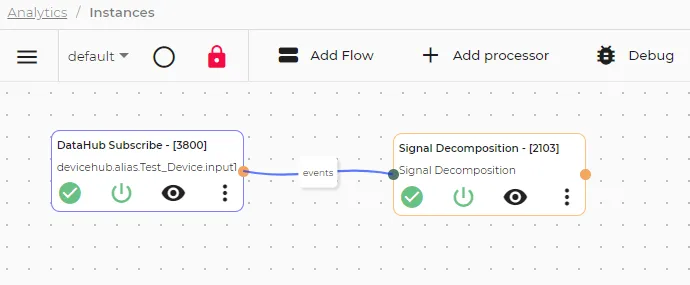
Click the View icon in the Signal Decomposition processor to view the output values.
The output shows the data with the trend removed at 166.05, a seasonal component of 131.78, and a positive trend in the data at 26.16.
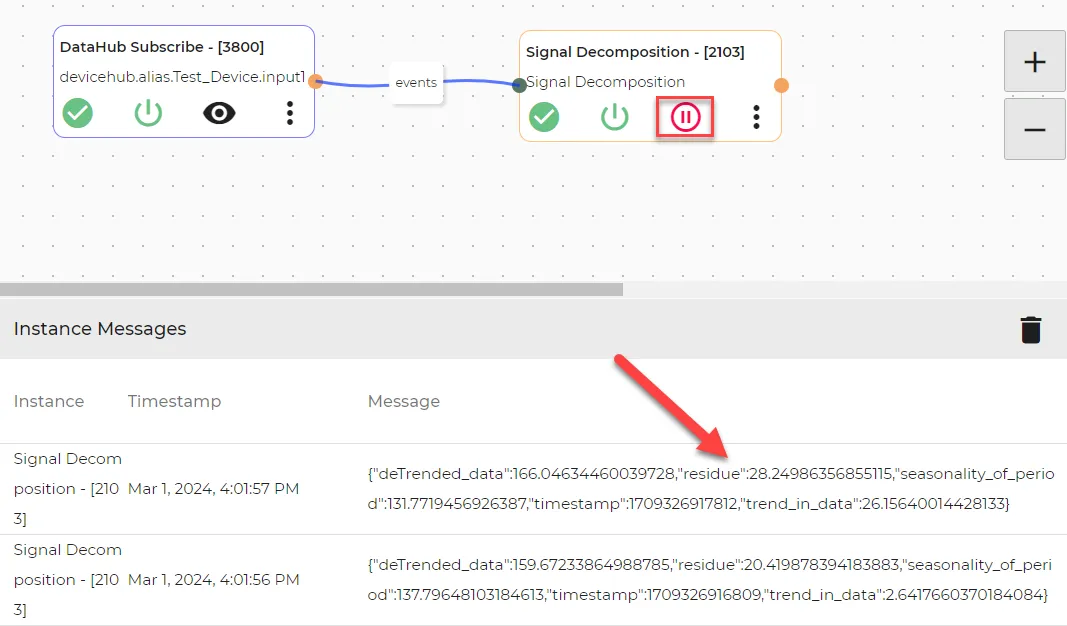